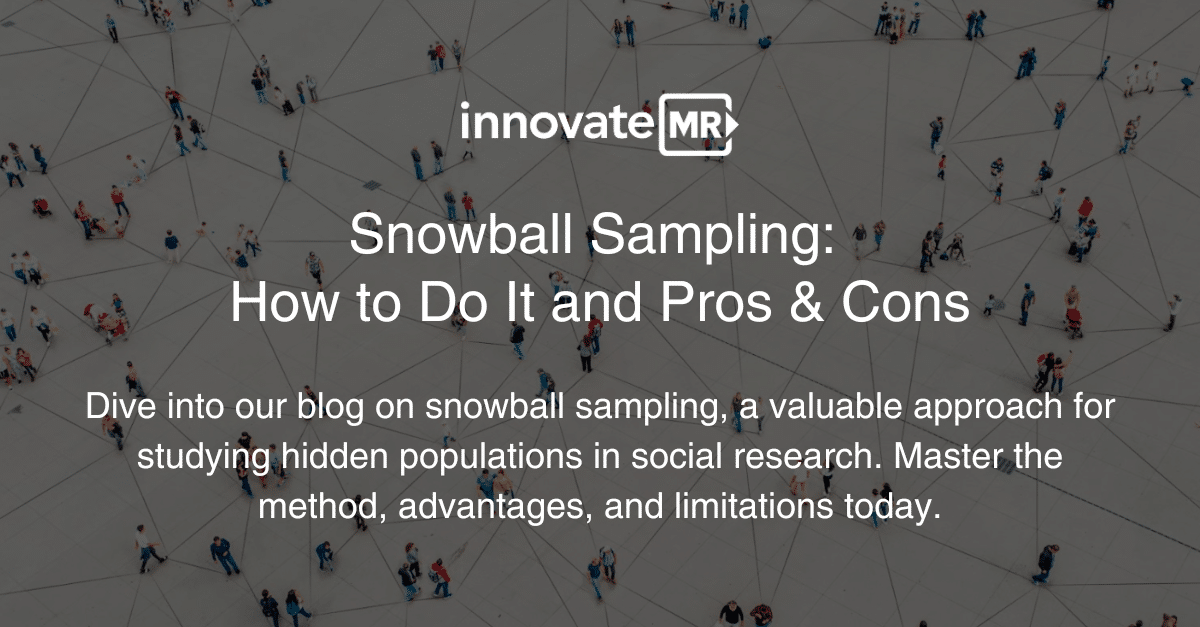
Snowball sampling remains one of the most effective non-probability sampling methods used in social science research today. Its ability to identify and recruit participants from hard-to-reach populations—those not easily accessible via traditional recruitment methods—makes it invaluable for studying sensitive topics or marginalized groups. As we progress into 2024, the snowball sampling technique has evolved alongside technological advancements, such as artificial intelligence (AI), which now plays a pivotal role in optimizing participant recruitment. In this detailed guide, we’ll explore how to conduct snowball sampling effectively, examine its pros and cons, and discuss how companies like InnovateMR can integrate cutting-edge technology to streamline the process.
What is Snowball Sampling?
Snowball sampling is a chain-referral, non-probability sampling technique where existing study participants recruit future subjects from their own social networks. This method allows researchers to tap into communities that might otherwise be inaccessible, whether due to privacy concerns, mistrust of outsiders, or the stigmatized nature of the population being studied.
For example, studying communities affected by rare medical conditions can be particularly challenging. Snowball sampling helps overcome these barriers by leveraging trust within these networks, enabling researchers to gather high-quality data from participants who might otherwise be reluctant to engage.
How to Conduct Snowball Sampling in 2024
Here’s a step-by-step guide to executing the snowball sampling method with modern updates, incorporating technology and ethical considerations.
1. Identify Your Population of Interest
Start by defining the specific population or community that you want to study. This group should share a characteristic or experience that is central to your research objectives. For instance, you may be interested in exploring the lived experiences of refugees, survivors of domestic violence, or people diagnosed with a rare illness.
Incorporating AI tools like predictive analytics can help refine your initial population selection by providing data-backed insights into trends and demographics. These tools, offered by research companies such as InnovateMR, can optimize your recruitment by identifying key characteristics that might help in more accurately defining your target population.
2. Recruit Initial Participants (Seeds)
Once the population is identified, you need to recruit a small set of initial participants, known as “seeds.” These individuals act as the starting point for your snowball sampling chain. Recruitment can be done through community organizations, social media platforms, personal networks, or third-party research platforms like InnovateMR, which can facilitate access to niche populations.
Incorporating AI in recruitment can make this process more efficient. AI-driven algorithms can analyze social media patterns or community data to find individuals who meet your criteria. Additionally, these algorithms can help minimize bias in seed selection by ensuring diversity and representation within the initial group.
3. Request Referrals from Initial Participants
Once your seeds are established, the next step is to ask them to refer others from their social networks who also meet your research criteria. Providing clear guidelines to your initial participants ensures that they refer candidates who are relevant to your study. Researchers should also consider using digital platforms to make this process easier—online survey programming tools can allow participants to directly invite others to join, speeding up the recruitment chain.
AI can further optimize this stage by analyzing referral patterns. It can identify potential oversampling or biases based on demographic data, helping you adjust your referral strategy as needed to ensure a more representative sample.
4. Repeat the Process
This referral-based recruitment process continues until you reach the desired sample size for your research. At this stage, AI’s role becomes critical. Tools like machine learning models can analyze participant data in real-time, providing insights on whether the sample is diverse or whether any adjustments are needed. This reduces the likelihood of sample bias and enhances the quality of your data.
By leveraging InnovateMR’s advanced technology platform, researchers can automate many aspects of this process, ensuring that they collect accurate, high-quality data with minimal manual intervention.
Pros and Cons of Snowball Sampling
Like any sampling technique, snowball sampling comes with its own set of benefits and drawbacks. Understanding these will help researchers determine if this method is the right choice for their study.
Pros:
Access to Hard-to-Reach Populations: Snowball sampling is ideal for populations that are difficult to access, such as individuals involved in illegal or stigmatized activities. Leveraging referrals from trusted members of the community helps establish rapport, resulting in more accurate and honest data.
Cost-Effective: Snowball sampling is a cost-effective alternative to more traditional recruitment methods, such as mass advertising. It primarily relies on personal referrals, which eliminates the need for expensive recruitment campaigns.
Efficient in Exploratory Research: For exploratory studies, snowball sampling allows researchers to quickly gather qualitative insights from under-researched populations. This method is particularly valuable when dealing with groups that are underrepresented in existing academic literature.
Enhanced by AI: The inclusion of AI-driven tools can make snowball sampling more effective by streamlining participant recruitment and ensuring a more representative sample. These tools can also provide real-time analytics to help adjust recruitment strategies as needed.
Cons:
Sample Bias: Snowball sampling’s reliance on referrals can lead to biased samples, as participants tend to recommend individuals who share similar characteristics. This can result in a lack of diversity within the sample, skewing the results.
Difficulty Estimating Sampling Error: Since snowball sampling is non-probability-based, it is challenging to estimate sampling error or determine the statistical significance of the data. This limitation makes it less reliable for studies that require rigorous quantitative analysis.
Limited Generalizability: Because the sample is drawn from personal networks, it may not be representative of the larger population. Consequently, the findings may not be applicable to other groups, limiting the study’s generalizability.
Ethical Concerns with AI: While AI can enhance recruitment, it’s important to be mindful of ethical considerations. Ensuring participant privacy and data security must remain a priority when using AI for snowball sampling, especially in sensitive research areas.
AI’s Role in the Future of Snowball Sampling
As we move further into the digital age, AI is poised to revolutionize how snowball sampling is conducted. Here’s how AI can shape the future of this method:
Automated Participant Identification: AI can analyze social media data, online communities, and other digital footprints to automatically identify potential study participants, drastically reducing the time required for recruitment.
Bias Reduction: AI algorithms can be programmed to detect patterns that might indicate sample bias, such as the overrepresentation of certain demographics. By alerting researchers to these patterns early in the process, AI can help ensure that samples are more representative.
Data Accuracy and Integrity: AI-driven analytics can process large amounts of data in real-time, allowing researchers to monitor the quality of their data and make adjustments as needed. This enhances the reliability of the findings, even in non-probability-based sampling methods like snowball sampling.
Enhanced Data Security: As AI becomes more integrated into research, ensuring participant privacy and securing sensitive information will become even more critical. Companies like InnovateMR are already at the forefront of incorporating AI in a responsible, ethical manner that prioritizes data security.
InnovateMR’s Role in Optimizing Snowball Sampling
InnovateMR offers cutting-edge solutions to streamline the snowball sampling process. Whether you’re conducting exploratory research or looking to target hard-to-reach populations, InnovateMR can support your efforts through technology-driven tools and expert knowledge. By integrating AI into participant recruitment, InnovateMR ensures that researchers can access diverse, high-quality samples while maintaining ethical standards and data security.
Conclusion
Snowball sampling continues to be a vital tool for social science researchers, especially when studying sensitive or hard-to-reach populations. As technology evolves, AI’s influence on snowball sampling is only set to grow, providing opportunities to optimize recruitment and improve data quality. Researchers can rely on partners like InnovateMR to provide the expertise and technological infrastructure needed to navigate the complexities of snowball sampling in 2024 and beyond.
About InnovateMR – InnovateMR is a full-service sampling and ResTech company that delivers faster, quality insights from business and consumer audiences utilizing cutting-edge technologies to support agile research. As industry pioneers, InnovateMR provides world-class end-to-end survey programming, targeted international sampling, qualitative and quantitative insights, and customized consultation services to support informed, data-driven strategies, and identify growth opportunities. Known for their celebrated status in customer service and results, InnovateMR combines boutique-level service with extensive global reach to achieve partner success.